Taking customer service to the next level with VirtualHumans and Large Language Models, a new age of virtual employees.
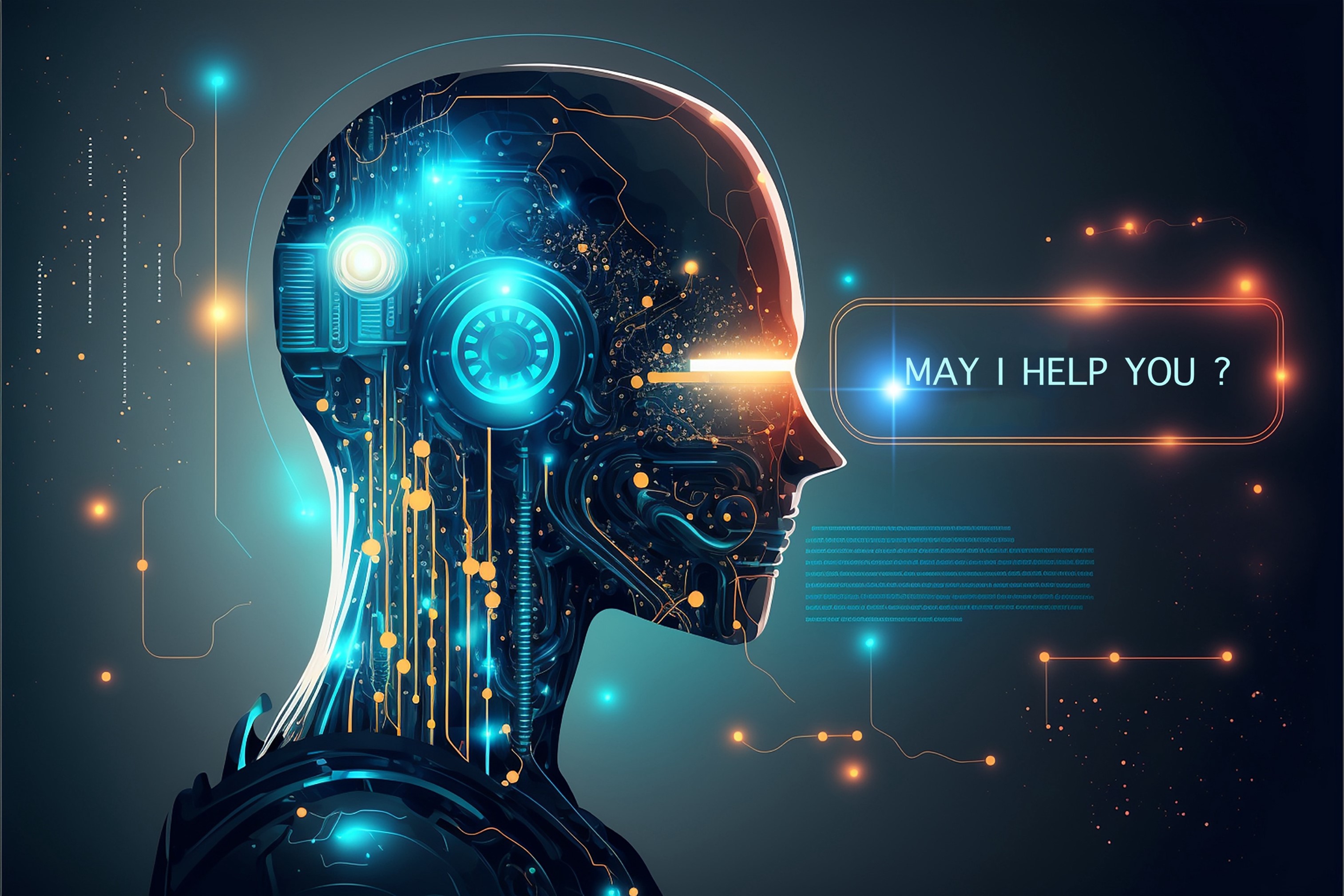
OpenAI has introduced generative AI to the masses with the introduction of ChatGPT, and it has become nothing short of a cultural phenomenon. This will be most people's first generative AI experience, but it won’t be their last. In its many forms, generative AI is set to transform countless aspects of business experiences and personal lives. Even more exciting is that when multiple generative AI models are combined, more powerful capabilities and experiences are enabled.
Generative AI refers to AI systems that are capable of creating new data or content, such as images, videos, music, or text, that is original and does not exist in the training data. AI image generators produce realistic images, while text-to-speech converters transform the written text into natural-sounding speech.
First, the answer to a general question, “What is generative AI?”
Let’s go to the source of all the buzz and ask ChatGPT to define generative AI. It quickly responds with, “Generative AI, or Generative Artificial Intelligence, refers to a type of artificial intelligence that is capable of creating new data or content, such as images, videos, music, or text, that is original and does not exist in the training data.”
This blog highlights the possibilities enabled when combining video generation models of DeepBrain AI and text generation capabilities of Large Language Models (LLM).
Before we talk about where we’re going, let's quickly recap where we are and what we have achieved to date. While generative AI is still in its infancy, and there’s no telling where the technology will take us, DeepBrain AI is already working with customers across multiple industries to support customer service initiatives through the deployment of its AI Avatar technology. Taking the financial industry as an example, DeepBrain AI has partnered with a number of institutions to deploy AI Human enabled kiosks and mobile apps to provide enhanced customer service capabilities for clients such as KB Bank, Shinhan Bank, Woori Bank, Hana Bank, NongHyup Bank, and Samsung Securities.
The AI Human solutions from DeepBrain AI already provide a natural conversational, humanistic interface for answering common questions, giving market updates, and handling basic transaction services. Meanwhile virtual assistants and virtual humans for banking show continued growth as banks look for more ways to increase efficiency and digitize their services while continuing to offer that personal feel provided by our AI avatars. According to Allied Market Research, the global virtual assistant market in the banking is expected to reach $11.2 billion by 2031.
However, while they are a powerful tool, they need to be tightly managed. They are not fire and forget solutions. At least not yet. Behind the scenes, all the answers, market updates, and chatbot experiences have been meticulously prepared by analysts, customer experience professionals, and developers providing these AI Humans with a fixed knowledge base and script to stick to, ensuring a reliable, repeatable, on-message customer journey.
But what happens when an AI Human is paired with a Large Language Model (LLM)?
In short – it can ‘flip the script.’ And not necessarily in a good way. While pairing a virtual human with an LLM like ChatGPT creates an exciting demo and a fun, futuristic experience, ChatGPT is still not suitable for serving clients or staying on message for a corporation.
It has been well-documented that ChatGPT can be unpredictable and even just plain wrong. ChatGPT even has a disclaimer on its website: “ChatGPT may produce inaccurate information about people, places, or facts.”
Furthermore, responses to questions often return with caveats “I'm sorry, as an AI language model, I do not have access to real-time data or the ability to browse external sources to provide you with the latest information. My knowledge cutoff is up to September 2021, and I cannot browse the internet.”
So, probably not the best candidate for a brand representative for a virtual human.
However, different industries are starting to understand the power of these LLMs when paired with their industry-specific data, which could start to change the sophistication and capabilities of these LLMs in the future.
By way of a recent example, on March 30, Bloomberg released a paper on its own LLM, BloombergGPT—the first reported example of an LLM explicitly trained for the finance domain. BloombergGPT is a powerful new model that can effectively summarize complex financial topics with headline generation, automatically generate Bloomberg Query Language (BQL) code to retrieve stock market data, and answer questions related to the financial world with higher accuracy than competitive models.
Pairing a virtual human with a domain-specific LLM like BloombergGPT opens a new world of possibilities. For starters, customers will be able to have natural conversations with an AI Human that is approaching the capabilities of a full-fledged virtual employee.
Looking beyond BloombergGPT, it is easy to imagine a future where most companies have a custom LLM to represent their brand and interact with customers. Maybe DisneyGPT learns how to interact like a Disney employee? Or NikeGPT knows all about every shoe and sports coaching? A future with industry-specific and brand-specific agents paired with an AI Human that becomes the virtual face and voice of the brand across all the digital touchpoints, web, mobile, and metaverse. Combining the video generation models of DeepBrain AI and text generation capabilities of Large Language Models takes virtual humans opens a new world of possibilities for serving customers with AI powered virtual agents.